When you’re running an ecommerce business, it’s important to know as much about your customers as possible.
You want to know who they are, what they’re interested in, how they’re interacting with your business, and, most importantly, what they’re buying.
Collecting all this information is vital if you want to optimise your business and increase your sales – but unless your superpower is data collection and interpretation, you’ll need help from your MarTech stack to gather and then make sense of this intel.
Two tools that many e-commerce businesses commonly rely on are audience engines and recommendation engines.
In this article, we will be comparing the two tools and providing examples of how they can be used in an e-commerce setting.
What Is an Audience Engine?

Audience engines, sometimes referred to as predictive analytics, seek to create meaning from user data and predict future customer behaviour. Think of this tool as your all-seeing crystal ball.
Audience engines (like QUIN) are focused on figuring out the intentions of your customers in real time. By interpreting user behaviour and understanding what shoppers are most likely to do next, audience engines can help you to nudge more shoppers along the funnel.
For example, if your audience engine knows that a shopper is likely to leave your page, that could trigger an offer that would tempt them to stay on page and complete their purchase.
Or, if your audience engine is integrated with your recommendation engine, it could trigger the recommendation engine to serve up a personalised suggestion for that consumer, based on the type of product they are looking for.
What Are Recommendation Engines?
Recommendation engines are a type of rule-based software. They suggest products and content for customers as they’re browsing.
This is commonly seen on large platforms such as Netflix, Spotify, and Amazon. Using browsing data, they see what kind of products, content, or music your visitors are looking for and suggest associated products.
Looking for running shoes? Take a look at these Nikes. Browsing for a murder mystery? Try these James Paterson books.
Importantly, the main aim of a recommendation engine is to promote existing and new products to customers in line with their search history. They don’t try to analyse the specific behaviours of the customers themselves and they don’t allow for changes in behaviour. That running shoe customer from three months ago likely has their new kicks. That book worm may already have a pile of books to read on their bedside table. A recommendation engine can’t account for those changes.
Recommendation Engine vs. Audience Engine
Although audience engines and recommendation engines are both important platforms for ecommerce businesses to use, they shouldn’t be confused with each other.
They are two very different pieces of technology that serve different purposes. That said, if you put an audience engine like QUIN at the centre of your MarTech stack, an integrated recommendation engine can make a clear contribution.
The important difference is:
· Audience engines are used to derive meaning from data and predict the future behaviours of customers.
· On the other hand, recommendation engines will use existing data to provide suggestions on different products or content ideas that can be promoted to customers. Think of a recommendation engine as being more of an immediate product suggestion tool, rather than a predictive behavioural analysis asset.
Examples of Audience and Recommendation Engines in Use

Both audience and recommendation engines can help you to increase sales and enhance the customer experience.
One example of a recommendation engine in use is Amazon. The online shopping destination wanted to increase its average order value. It was able to do this by employing recommendation engine technology to help buyers discover new products, therefore encouraging them to buy more.
The goal was to encourage customers to buy items that they didn’t even realise they wanted before it was promoted to them. Shoppers on Amazon will now see recommendations on what Amazon thinks they will want to purchase. Then, when they add this item to their basket, they will also see another range of products that other similar customers have also purchased.
Amazon has been able to successfully include relevant product recommendations at every point in its customer journey, providing more opportunities to upsell and cross-sell.
Similarly, it’s common for ecommerce brands to use audience engines to personalise the customer experience.
For example, brands can use data such as abandoned carts, products with no sales, page views, bounce rate, total sales, and checkout values to understand how their customers are interacting with their site. They can also gain information on the devices customers use to browse, where they are coming from, and how many different pages they visited on the website.
Brands like Airbnb use audience engines to create their dynamic pricing strategy. They analyse data such as the popular seasons, popular days of the week for booking, nearby events, etc. Then, they will use this data to suggest the optimal pricing to landlords.
Final Thoughts
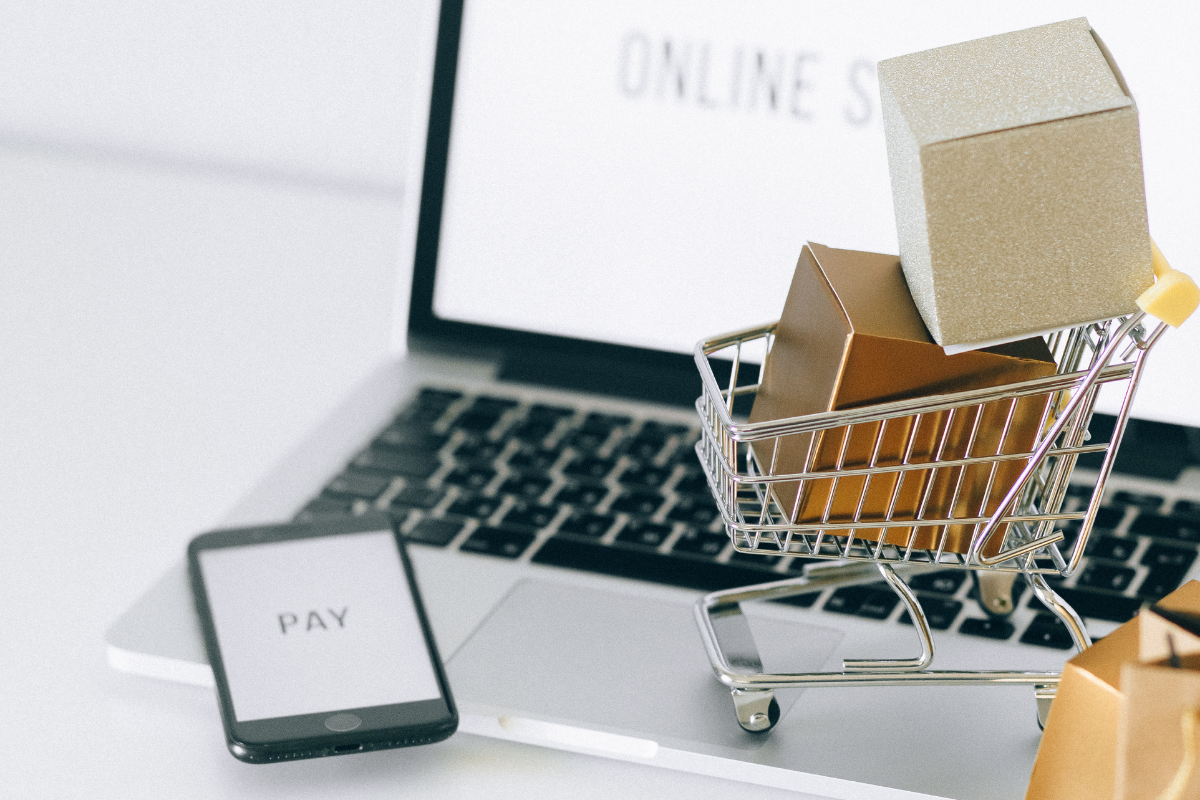
Audience and recommendation engines are two very different, yet important tools for digital retail.
Audience engines use predictive analytics and behavioural data to provide personalised experiences to customers, whereas recommendation engines use this data to come up with relevant product and content recommendations.
Together, they can help ecommerce brands build brand loyalty, improve marketing strategies, create more personalised experiences, and maximise online sales.
To Read the whole post