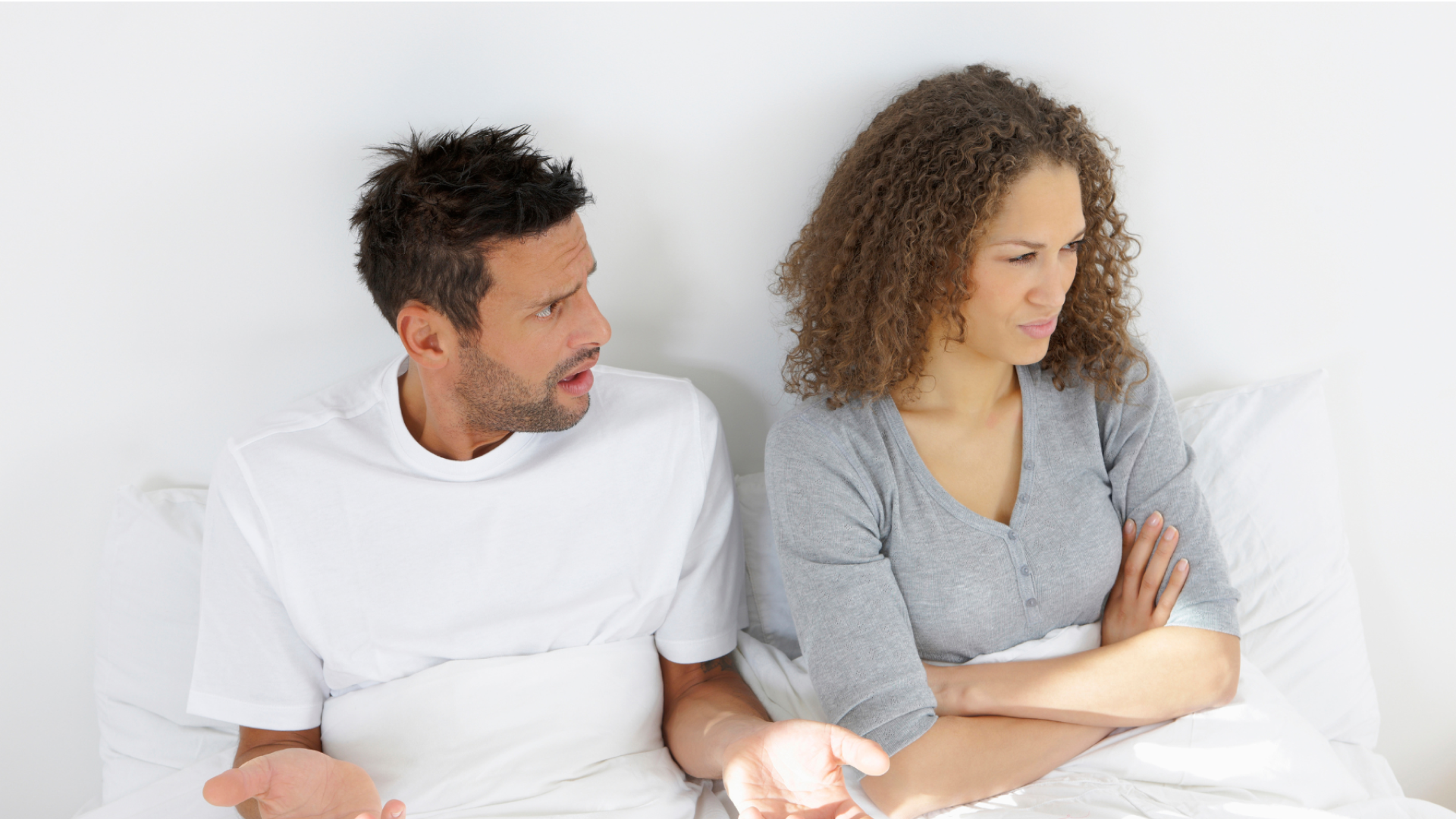
We live in a world overflowing with data. With every click, swipe, and purchase, we leave behind a breadcrumb trail businesses scramble to follow, eager to personalize our digital experiences.
Yet without context, all that data can become misleading noise – turning personalization into guesswork that can do more harm than good. It’s a problem we’ve seen time and again.
So, in this post, I’ll share four real-world stories of personalization gone wrong to highlight why context-aware, ethical data usage isn’t just a ‘nice to have’ – it’s essential for building trust and delivering relevant, respectful customer experiences.
1. The pitfalls of household-based targeting
Let’s start with a classic CRM misstep – one where good intentions collide with outdated assumptions.
While managing ongoing email communications for a large travel brand, I noticed that their system was still built around a legacy idea: the concept of “households.”
Whenever someone booked a trip, anyone connected to the same address, past bookings, or payment details was grouped together and treated as if they shared the same preferences and consent.
Most of the time, this passed unnoticed. But one case exposed just how fragile that logic was.
A man contacted the brand, alarmed by a flood of promotional emails about a luxury trip to Mauritius – a trip he had no memory of booking. Fearing identity theft, he raised the alarm.
When we traced the records, the truth surfaced. Unfortunately, his partner had booked the holiday using their shared payment details but didn't intend to include him in the experience. Instead, she planned to take her boyfriend...
The CRM didn’t recognize this nuance and simply lumped them together, targeting both with identical emails. As you might imagine, the fallout was bigger than hitting the 'unsubscribe' button. The misdirected emails became central to legal proceedings as the man initiated divorce proceedings. Eventually, every message sent through the CRM became part of the evidence.
Lessons learned
This story shows how even well-meaning systems can miss the nuances of real life. Personalization without awareness of context or consent doesn't just risk irrelevant messaging – it can compromise privacy, strain customer trust, and, in this instance, lead to much larger personal fallouts. It’s a sharp reminder that customers aren’t patterns or assumptions. They’re individuals, and personalization strategies need to treat them that way.
2. The gas station loyalty loophole
This story takes us overseas to Turkey. At their gas stations, attendants routinely filled customers’ cars – a standard practice that, at first glance, seemed harmless.
However, when I analyzed their loyalty program data, a disproportionate share of cashback rewards kept going to the same small group of people, raising suspicions about potential misuse.
It turned out that some attendants were swiping a loyalty card – but just not the customer’s. Instead, they used their own or one registered under a relative’s name. Over time, this simple trick allowed their families to accumulate significant cashback rewards and perks.
From a purely data-driven perspective, the loyalty program couldn’t distinguish between a genuine, high-value customer and an employee gaming the system. As a result, the company was unknowingly misallocating rewards, leading to budget losses and a distorted view of customer engagement.
Lessons learned
It’s easy to collect data – but without the right systems to make sense of it, that data can send you in the wrong direction. Businesses need tools that spot patterns and catch when something feels off before it becomes a bigger problem. Otherwise, rewarding the wrong behaviors and wasting your precious marketing budget is too easy.
3. The diapers and breast pumps blunder
Let’s move to e-commerce, where targeting misfires are often the most visible – and sometimes, the funniest.
For example, a male friend of mine once bought a pack of diapers online as a gift for a friend’s newborn baby. A week later, he added a few baby toys to his cart – another thoughtful gesture.
But fast forward a month, and his inbox and ad feeds were flooded with promotions for breast pumps. The algorithm had quickly (and incorrectly) labeled him as a new Mom, relying solely on a couple of purchases. It hadn’t considered the bigger picture – was he actually part of the target demographic? Was this a one-off purchase or part of a larger pattern?
In this case, the algorithm failed to consider the broader context of his purchases, leading to irrelevant (albeit funny) recommendations.
Lessons learned
This mix-up is a common e-commerce headache. When systems jump to conclusions too soon, people get shown things they don’t care about. They tune out, and the connection’s lost. The fix? Recommendations should change as customer behavior does in the moment – staying flexible and adjusting in real-time to meet the shopper where they're at.
4. The affluent customer – That wasn’t
Last but by no means least, here’s one of my favorite data stories underscoring how dangerously misleading assumptions can be.
A few years ago, while working with a major telecom company, I was tasked with helping segment customers based on how much they spent on their monthly phone bills. The goal was to identify "affluent" customers and create exclusive products and perks tailored for them – think premium packages and even offers like free parking at Harrods.
At first glance, it seemed logical. Customers with high bills must be wealthier, right?
Wrong.
We soon found that a big bill doesn’t necessarily equate to a big bank balance. We randomly selected several high-bill customers and called them directly to understand who they were.
Here’s what we found:
Many were local truck drivers, racking up large phone bills thanks to roaming charges. Others were taxi drivers, constantly on the move. But, a surprising number were sex workers, whose lifestyle naturally resulted in high phone usage. Needless to say, none of them fit the millionaire demographic the company had envisioned!
Lessons learned
Data without context doesn’t equal insight. Making assumptions about customers based purely on spending, location, or isolated behaviors can spectacularly backfire.
How to avoid context-blind personalization
Want to avoid being the brand that accidentally fuels divorce proceedings or promotes breast pumps to bachelors? Here’s your checklist:
- Treat customers as individuals, not static data points: Don’t lump people together based on outdated assumptions. Your personalization strategy should reflect real people, not database shortcuts.
- Use real-time behavioral signals, not just purchase history or demographics: Tracking how customers behave right now allows you to tailor real-time recommendations that meet their needs. Context changes fast, and your personalization should change with it.
- Respect privacy and consent: Personalization should always feel helpful and never invasive. Context-aware personalization means knowing when not to cross the line. Ensure customers are in control of how their data is used and never rely on inferred relationships without explicit consent.
- Continuously learn and refine your engagement strategies: Your customers aren’t standing still, so why should your models be? Use AI systems that evolve with each interaction, spotting patterns, anomalies, and shifts as they happen – before they spiral into bigger issues.
Pro tip: If your current tools can’t tick these boxes, it’s time to upgrade to something that can. (Hint: We know a good place to start.)
At Quin AI, we help businesses avoid the pitfalls mentioned above through real-time, ethical personalization based on live behavioral insights – no guesswork or assumptions, just relevant, respectful customer experiences.
Curious to see how Quin AI can help you deliver personalization that gets it right every time? Book a demo today.
To Read the whole post