
It’s been 42 years since the first eCommerce marketplace opened its virtual doors. Today, eCommerce is coming of age. We’re entering an era whereby the use of advanced AI technologies will no longer be optional. We’re not talking about warehouse robots or chips implanted in human brains – rather, we’re considering how vital AI is to managing operations and the critical role this technology now plays in providing high quality, personalized services, and experiences.
The good news? You haven’t missed the boat. Relatively speaking, AI adoption is still in its infancy. That means there are significant opportunities in this transition. What’s more, it pays to get in on the ground floor because as AI technologies advance, those opportunities will only increase.
AI, like all other technologies, is not perfect. It does come with flaws that can impact businesses just as easily as it can help them. One of those flaws is the presence of bias.
While by no means prolific, there is evidence to suggest that AI algorithms may inadvertently introduce bias and discrimination [1].
These biases can manifest in various ways including in gatekeeping or offering unequal access to products or services based on race, gender, or socioeconomic status. As a business leader, you need to be aware of the potential for bias. Awareness is your superpower. If you’re aware of it, you can take steps to combat it. If you remain in blissful ignorance, it could well be your Kryptonite.
The Pitfalls of Misguided Algorithms
It’s common practice for eCommerce companies to collect data about their customers. Knowing how they behave across multiple channels, what they like, what they buy and how often makes it easier to please them by better anticipating their needs in future. Simple, right?
It should be.
The truth is that collecting demographic and historical information about customers can mislead AI algorithms. It can inadvertently lead them down the path of making generalized, biased conclusions. Those conclusions can have real world implications, ranging from the loss of customers [2] to legal issues when assumptions damage well-being [3].
Awareness is the first line of defence so having a working knowledge of how AI technologies can demonstrate bias is the first step.
Friends, Family, and the Phantom Consumer:
Grandpa George is bombarded with baby clothes. That’s not because he’s about to be a new dad. It’s because he and his daughter (who just became a mother) share a computer. This case of mistaken identity can cause confusion. At the more dramatic end of the scale, it can also alienate customers and mean what should be powerful personal recommendations become a missed opportunity. That missed opportunity means you’re losing out on revenue.
While collecting user ID and device data is widespread, it’s important to remember that people commonly share devices and accounts. The AI algorithm can't identify the family member sitting in front of the computer, so there’s potential for bias or incorrect assumptions to creep in.
The Invisible Majority
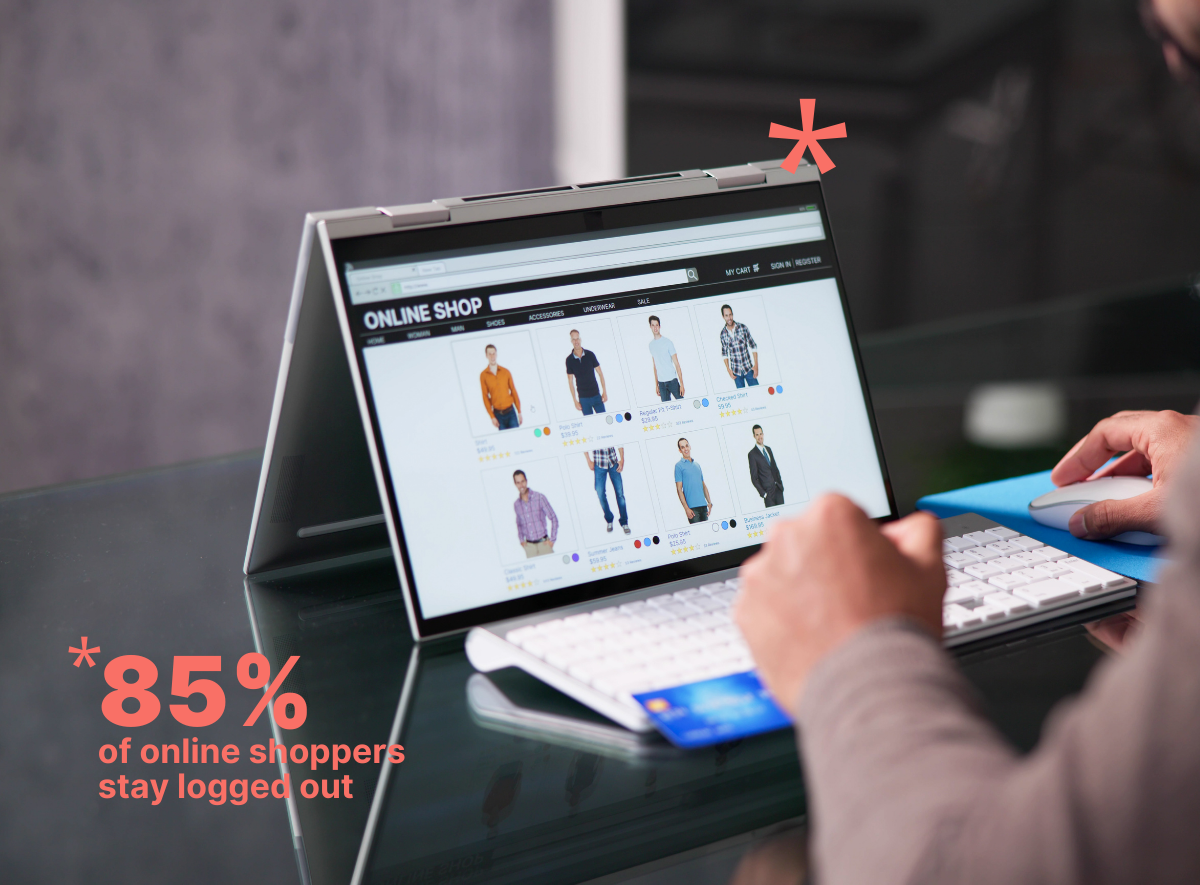
Did you know that more than 85% of online shoppers stay logged out? [4]. If your AI algorithms rely on demographic or historic data to build a picture of your consumers, there’s a big question mark over that 85%. To compensate for that unknown, AI develops generalized customer journeys. That’s a problem because with no specifics to go on, AI can’t live up to its potential to serve personalized and meaningful experiences. It’s like blind dating in a power cut with no light or sound.
The Stereotypical Algorithm
AI algorithms can reinforce stereotypes if they’re trained with biased data [5]. Algorithms which run on stereotypes can discriminate and that makes customers feel invisible and unwelcome. That’s enough to lose the sale [6][7]. Relying on outdated assumptions also means that customer diversity is ignored – something which also strikes a blow to your bottom line and to your reputation.
Let’s say your training data suggests that kitchen appliances are mostly bought by women. The AI might start recommending kitchen appliances primarily to women, reinforcing the stereotype that cooking is primarily a woman’s job. This could make male customers who enjoy cooking feel invisible or unwelcome. The end result is they go elsewhere, and you lose the sale.
A Changing World
We might assume that tastes and expectations stay constant, but the reality is that we all change and grow over time. If your AI stack only works with historical data, it can’t evolve with your customers.
Recommending the same content or products year after year isn’t just frustrating. When your recommendation engine misses the mark, it’s easy for shoppers to assume that you don’t have what they need and take their money elsewhere.
Let’s put this into context. Imagine you’re an online electronics store using AI to recommend products. One of your loyal customers purchased gaming equipment many moons ago. More recently, they’ve started a new job or embarked on a new career. Instead of gaming PCs, they need a Mac that can handle graphic design, a printer, a scanner, and a photo copier.
If your AI doesn’t understand that young gamer has grown into an ambitious entrepreneur, it will continue to recommend gaming products based on that past data. In the real world, it’s failing to meet that shopper’s current needs. Your budding entrepreneur is frustrated, time poor and not getting what they need. No sale.
What Are the Real World Consequences of a Biased AI Algorithm?
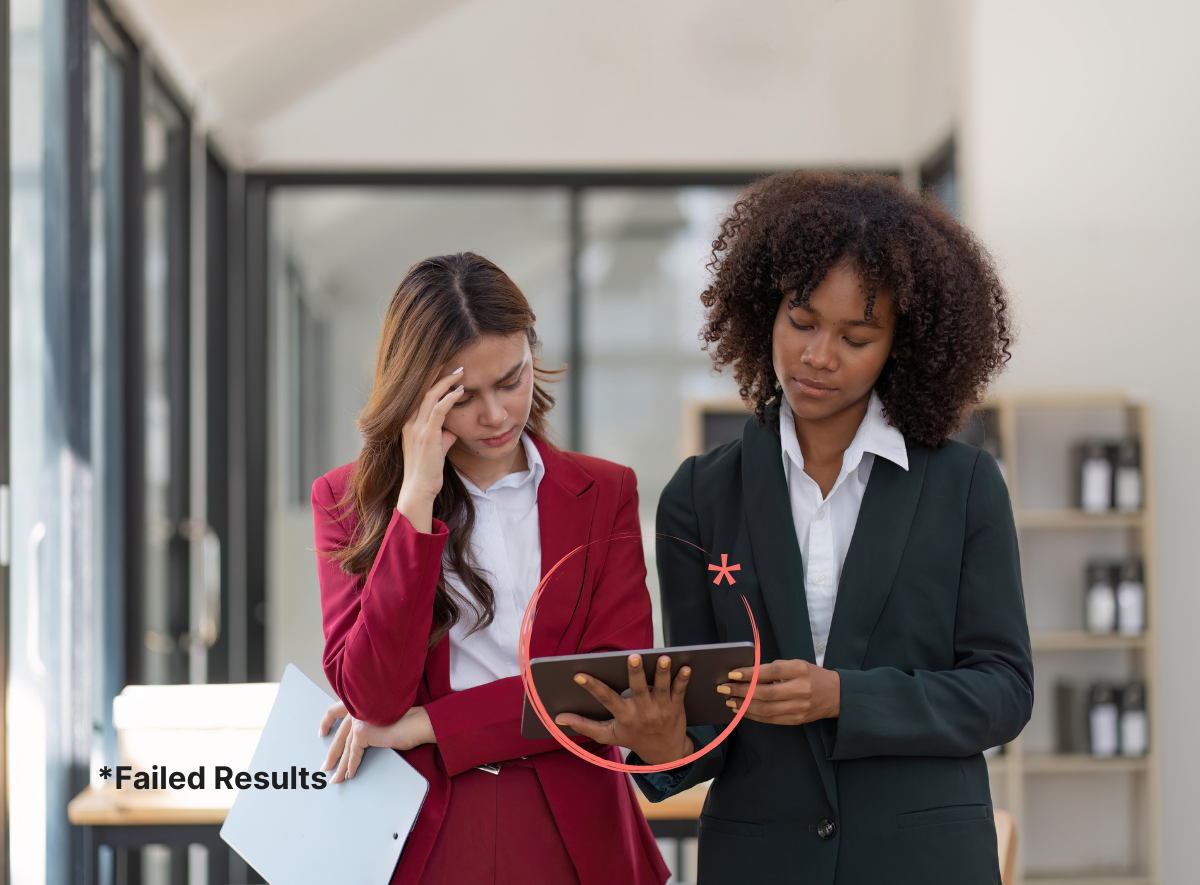
If your AI algorithms introduce bias and discrimination, you risk alienating huge chunks of your demographic. More seriously, you could inadvertently be giving unequal access based on metrics such as race and gender. The fall out from that isn’t just a cohort of frustrated shoppers wondering why your site is suggesting entirely inappropriate items.
Consequences beyond those frustrated clicks include:
Significant Lost Revenue: Misinterpreted, misunderstood, or misrepresented customer intentions can lead to unhappy customers and lost revenue.
Eroded Trust: Customers who feel unfairly targeted or ignored may not return, taking their loyalty and spending power elsewhere.
Damaged Reputation: Negative reviews and poor word-of-mouth can spread quickly, tarnishing your image, and deterring potential customers.
Increased Legal Risk: Discriminatory practices based on biased algorithms can lead to legal issues with regulatory bodies and consumers alike.
These consequences underscore the importance of addressing AI bias through fairness, transparency, and accountability.
Fighting Back against Bias
So, now you know that a problem could exist, how do you fight back against inadvertent bias?
Ethical Data: Alternative, unbiased, and ethical datasets – which are free of data that can potentially weigh towards a certain demographics or behaviour - can reduces bias. They do this by capturing realistic customer wants with no preconceived notions based on small data sets. This is a double edge sword because your best intensions could make the problem worse. Diversifying your data could lead to bias becoming more widespread, as collecting information about religion, race and other characteristics could run the risk of discriminating against those very shoppers you’re seeking to better serve.
Challenge Assumptions: Regularly auditing your AI models and learning algorithm strengths and weaknesses is good practise. This is one way to ensures fairness and stay on top of potential issues. Remember, correctly developed AI capabilities surpass individuals for understanding customers [8]. Consider introducing new paradigms to challenge assumptions at regular intervals.
Transparency: Explain how AI is being used across your digital channels to personalize the individual experience of each shopper. Being transparent about your use of AI – and why you’re using it – can help to build trust [9].
Conclusion
You can help your customers to feel valued by fighting bias. Acknowledging bias exists, implementing responsible practices, and embracing transparency transforms the user experience. It takes AI from the shadows, where it could be perceived as a biased oracle into a powerful, inclusive tool that strives to help and empower.
Facing similar issues, we created Quin's Audience Engine. It’s the first no-code deep learning platform using real-time behaviour to both understand and act on needs and intentions. We’ve made it our mission to make cutting-edge technology accessible. Find out more today.
Sources:
[1] What Do We Do About the Biases In AI? – Harvard Business Review. https://hbr.org/2019/10/what-do-we-do-about-the-biases-in-ai
[2] The Cost of AI Bias: Lower Revenue, Lost Customers. https://www.informationweek.com/data-management/the-cost-of-ai-bias-lower-revenue-lost-customers
[3] How AI model bias impacts trust | Deloitte Insights. https://www2.deloitte.com/us/en/insights/focus/cognitive-technologies/ai-model-bias.html
[4] Anonymous Visitors Are On The Rise: Is Your E-Commerce ... - Forbes. https://www.forbes.com/sites/forbestechcouncil/2023/02/28/anonymous-visitors-are-on-the-rise-is-your-e-commerce-business-ready/
[5] How to train your AI: Uncovering and understanding bias in AI algorithms. https://gender.stanford.edu/news/how-train-your-ai-uncovering-and-understanding-bias-ai-algorithms
[6] The Cost of AI Bias: Lower Revenue, Lost Customers. https://www.informationweek.com/data-management/the-cost-of-ai-bias-lower-revenue-lost-customers
[7] Shedding light on AI bias with real world examples - IBM Blog. https://www.ibm.com/blog/shedding-light-on-ai-bias-with-real-world-examples /.
[8] Clarifying Assumptions About Artificial Intelligence Before Regulating. https://academic.oup.com/grurint/article/71/4/295/6528412
[9] Building Transparency into AI Projects - Harvard Business Review. https://hbr.org/2022/06/building-transparency-into-ai-projects
To Read the whole post